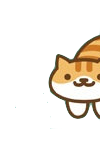

User Controls
🍬🍬Candy~Land🍬🍬
-
2024-08-07 at 3:52 PM UTC
Originally posted by Bradley
https://md5decrypt.net/en/Brainfuck-translator/
1995?,????????????35,000????,????????????????,????????????????????? ????????????????.????(Theodore Kaczynski)??,?????"?????"? 1978 ??1995 ??,??????????????????,??????????,???????????????????,??3 ????23???? ??????"?????",????????????????
?????????????????????,?????????,??????? ????????????????? ???????????,????????????,??????????????????????????,?????????????????????????????????? ????????????,?????????????????(???????,????????????Netflix ???,??????????????????)?????????????????
????????,????????????????????,?????????????????? ??????????????????????????????????? 27 ??,??????????????:
??????????????????? ???????????????????????????,????????????,????????,???????,??????????(????????????)?????????????? ????????????????
2018 ?,????????? Cambridge Analytica ???? Facebook ??,???????????????????? ?????????????????? ????????; WhatsApp ???????????????? ?????????????????,???????????? ????????????,??????????????????????????????????????????,???????????? ?????????????.???.?????????,????????,????????,?????? ??????,???????,???----?????????????????????????????????????????----?????????????????????????????????? ?????????????????????????????????????????????????????? ??????----?????????????????????----?????????????????? ????????
???????????????????K?,?2 ?18 ????????????????????? ?????????????????,???????????? ?????????,????????????????????? ??????,????,??????,?????,???????,??????????? ????????????????,????????????? ???????(????????)? ???????????????,??????????????(?????????,????,??)?
???????????? ??????????(??????,?????????????,?????),????????????? ???????????????,???????????????????????????----??????????????,???????????????? ???????????????,????????????,??"??????",????,???????????,?????????? ?????? ????????????? ????,??????,??????????(????????????????????????????)?
?????????????????????????????,??????????????? ?????????????????,?????????????????,??????????????----??????.K????????,???????????????? ???????????????
????????????????,?????????????????????????????; ??????????????????????? ??????????,?????????????????? ????????????????????,?????????????????????????????????????????????????
?????????,?????????? ? 1971 ?????????????????????,????????????????? ????????????????,??????????? ?????----????????,????----?????????????????????? ??????,??????????? 1990 ????????????????????????????????????????? ????????????????,?????????????,????????? ???????????? T ?? 90 ?????????????,????????????????????
???????????????????.???(Timothy McVeigh)???????,???????????????????????? ??????????????,???????????????,?????2b�??90�???????????�??????????????????????????????�?????????????????����???????????����??????�???????????????????�????????����?????????????????????????????????????????????????????5????????????????????????????????5???A????????c???cm???m???�?????????????�?????????????????�?????????????�???�????????????????�???????????????�?????????????�?????????????????????�????,???????????????????????????????????????????????????????_???????????a????k???????????????????????��????�?????��???????????????�?????????�???????????????????????�????????????����??????????????????????????????????????????????E????????IIivv????????????�????????????�????????????�?????????????�????????????????�??????????????�??????????????�???????;�??????????;�?????????;�??????????????;�???????????????????????�?????????�????????�??????????????????????????????????????H?????????????????H??????p?????????q??????�FC�??�???????????????�????????�????????????????�???????????????????,???????????,?????????????????,???????????????�???�??????????�?????????????????????�??????�????????:??????????????!????????Cc??o?????o????????????o???????????????????????y??????,???????????????????????,??????????????�?????????�??�Norbert�Weiner�???????????????????96Oo???:?????????????????????{????????????�??????????????�??????????�???�Jacques�Ellul�?????????????"???+964K????????????????T?t?????????????????????????t????????�??????�??????�???????????????�?????????????????????�????????�????????#?????????;#????????#?????????????/?????????????/???????????????O??????[??????????????????e????????????FC????????????:e??????????{???????????�??????�???????????????�??????????;�??????????????????????????�?????�????????�????�??�???????????�??�1971�?�???????????????????�1967�??�69�???????????????+???????7???9????aHerbert�Marcuse��???????????�UC�San�Diego��???????)????))????????3?????????Uu?u??????????}One�Dimensional�Man���� I???Ln??????????????????yyyy?�????????????�??????????��???????????????????????�??�????????????????????????????????????????:??????????????????????????????????????Aa????????????????a????????????????a??????????????????m????o???????o?????�1944�?????????:??????????????�???????????????????,?????????????????????????�????�?????????????�????�?????����????????�????
??
???????4?????????@?????????????????????????????????`???????????l?????????????????�????????????????????????????�???????????????????????????????????:???????????,??????????�??�???????????????????????�????????????�????????????????����???????????????????????????????????????????????????????????????????:???????????????????1????????????????????????????=??????????]??i???i??�??�?�??�??????????????�??????????????????????,??????�?????????????����???????????????����?????????�?????????�Lutz�Dammbeck��=?????????????????I??KKk??????????????????????????????k????????????w??????????????????????w???????????????????�???????????�????????????�????????????????�?????????????�???????????????:????????????�?????????�??�????????�?????????????�?????????????????????? -
2024-08-07 at 4:06 PM UTCComputational Life: How Well-formed,
Self-replicating Programs Emerge from Simple
Interaction
Blaise Agüera y Arcas† Jyrki Alakuijala† James Evans‡ Ben Laurie†
Alexander Mordvintsev† Eyvind Niklasson† Ettore Randazzo†
Luca Versari†
†Google, Paradigms of Intelligence Team and ‡The University of Chicago
{blaisea, jyrki, benl, moralex, eyvind, etr, veluca}@google.com
jevans@uchicago.edu
Abstract
The fields of Origin of Life and Artificial Life both question what life is and how it emerges
from a distinct set of “pre-life” dynamics. One common feature of most substrates where life
emerges is a marked shift in dynamics when self-replication appears. While there are some
hypotheses regarding how self-replicators arose in nature, we know very little about the general
dynamics, computational principles, and necessary conditions for self-replicators to emerge.
This is especially true on “computational substrates” where interactions involve logical,
mathematical, or programming rules. In this paper we take a step towards understanding
how self-replicators arise by studying several computational substrates based on various
simple programming languages and machine instruction sets. We show that when random,
non self-replicating programs are placed in an environment lacking any explicit fitness
landscape, self-replicators tend to arise. We demonstrate how this occurs due to random
interactions and self-modification, and can happen with and without background random
mutations. We also show how increasingly complex dynamics continue to emerge following
the rise of self-replicators. Finally, we show a counterexample of a minimalistic programming
language where self-replicators are possible, but so far have not been observed to arise.
K eywords Origins of Life · Artificial Life · Self-replication
1 Introduction
The field of Origins of Life (OoL) has debated the definition of life and the requirements and mechanisms
for life to emerge since its inception [ 1]. Different theories assign varying importance to the phenomena
associated with living systems. Some consider the emergence of RNA as the major turning point [ 2 ], while
others focus on metabolism or chemical networks with autocatalytic properties [ 3, 4]. The question of what
defines life and how it can emerge becomes necessarily more complex if we shift focus from “life as it is” to
“life as it could be”, the central question for the Artificial Life (ALife) community [ 5 ]. While searching for a
general definition of life, we observe a major change in dynamics coincident with the rise of self-replicators,
which seems to apply regardless of substrate. Hence, we may use the appearance of self-replicators as a
reasonable transition to distinguish pre-life from life dynamics [6].
Many systems involve self-replication. RNA [ 7], DNA, and associated polymerases are commonly accepted
self-replicators. Autocatalytic networks are also widely considered self-replicators [8]. Self-replicators are also
widespread in computer simulations by design. Most ALife experiments agents have predetermined methods
of self-replication, but several experiments have also studied the dynamics of lower level and spontaneous self-
arXiv:2406.19108v2 [cs.NE] 2 Aug 2024
Agüera y Arcas et al.
replication. Famously, Cellular Automata (CA) were created to study self-replication and self-reproduction [9 ].
Self-replicating loops with CA have been extensively studied [ 10, 11, 12]. A recent extension of CA, Neural
CA [13 ], can be trained to self-replicate patterns that robustly maintain interesting variation [14 ]. Particle
systems with suitable dynamical laws can also demonstrate self-replicating behaviors [ 15 ]. Neural networks
can be trained to output their own weights while performing auxiliary tasks [ 16 ] and they can be trained to
self-reproduce with meaningful variation in offspring [17]. Finally, self-replicators can exist on computational
substrates in the form of explicit programs that copy themselves, as in an assembly–like programming
language [18, 19], or a LISP-based environment [20], but this area of inquiry remains underexplored, and is
the focus of this paper.
Much research on OoL and ALife focuses on the life period when self-replicators are already abundant. A
central question during this period is: How do variation and complexity arise from simple self-replicators?
Analyses often take the form of mathematical models and simulations [ 21 ]. In ALife, researchers often focus
on selection for complex behaviors [ 22 ], which may include interactions with other agents [ 23 ]. Simulations
may include tens of thousands of parameters and complex virtual ecosystems [ 24 ], but they can rarely
modify the means of self-replication beyond adapting the mutation rate. The two most notable exceptions
use assembly-like languages as computational substrate. In Tierra [ 18], simple assembly programs have
no goals but are given time to execute their program and access and modify nearby memory. This causes
them to self-replicate and manifest limited but interesting dynamics, including the rise of “parasites” that
feed off other self-replicators. Avida [ 19 ] functions similarly: assembly-like programs are left running their
code for a limited time. They can also self-replicate, this time by allocating new memory, writing their
program in the new space, and then splitting. Avida adds a concept of fitness, since performing auxiliary
computation increases a replicator’s allotted execution time. Notably, both Tierra and Avida are seeded with
a hand-crafted self-replicator, called the “ancestor”. This puts them squarely into “life” dynamics, but still
allows for modification of the self-replication mechanism.
But how does life begin? How do we get from a pre-life period devoid of self-replicators to one abundant
with them? We know that several systems, initialized with randomly interacting primitives, can give rise
to complex dynamics that result in selection under pre-life conditions [6]. The OoL field has extensively
studied autocatalysis, chemical reactions where one of the reaction products is also a catalyst for the same
reaction, as well as autocatalytic networks (or sets), groups of chemicals that form a closed loop of catalytic
reactions [ 25]. Autocatalysis appears fundamental to the emergence of life in the biological world. Moreover,
autocatalytic networks arise inevitably with sufficiently distinctive catalysts in the prebiotic “soup” [ 26].
These have also been simulated in computational experiments [8, 27, 20, 28, 29, 30].
Fontana [20 ], for example, simulates the emergence of autocatalytic networks on the computational substrate
of the lambda calculus using LISP programs (or functions). Each element is a function that takes another
function as input and outputs a new function. Thus, a graph of interactions can be constructed which, on
occasion, gives rise to autocatalytic networks. Fontana also performed a “Turing gas” simulation, where a
fixed number of programs randomly interact using the following ordered rule:
f + g −→ f + g + f (g) (1)
Where f and g are some legal lambda calculus functions. To conserve a fixed number of programs, one of the
three right-hand side programs was eliminated using rule-based criteria. Aside from autocatalytic networks,
a very simple solution involves the emergence of an identity function i, yielding:
i + i −→ 3i (2)
This program has strong fitness, and it was often observed that the entire gas converges to the identity. This
can be considered a trivial replicator, which in some experiments is explicitly disallowed by constraint.
In [ 28 ], the authors use combinatorial logic to create an “artificial chemistry” founded upon basic building
blocks. Their system preserves “mass” (operations neither create nor destroy building blocks) and results in
simple autocatalytic behaviors, ever-growing structures, and periods of transient self-replicator emergence.
While lambda calculus and combinatorial logic are related to programming languages in general, they represent
distinct computational substrates. For example, creating RNA-like structures that can self-replicate arbitrary
payloads may involve different approaches, depending on the substrate. Biology is steadily furthering insights
regarding the conditions under which complex replicators such as RNA and DNA could have arisen and
under which conditions. This question is underexplored for the general case, especially on computational
substrates. Given recent advances in Artificial Intelligence, computational substrates could very well form
the foundation for new forms of life and complex, evolving behavior.
In this paper we focus on computational substrates formed atop various programming languages. Here we
highlight some of the most relevant previous investigations of the pre-life period on such substrates [29 , 30 , 31 ].
2
Agüera y Arcas et al.
In all of these investigations, and in ours as well, there is no explicit fitness function that drives complexification
or self-replicators to arise. Nevertheless, complex dynamics happen due to the implicit competition for scarce
resources (space, execution time, and sometimes energy).
In Coreworld [ 29, 30], the authors explore the substrate of programming languages with multiple programs
executed in parallel and sharing the instruction (and data) tape. Programs consume a locally shared resource
(energy) for executing each operation. The authors perform different runs where they observe complex
dynamical systems resembling the pre-life period hypothesized in biology and observed in our experiments
as well. In Coreworld, large structures appear alongside inescapable self-loops. Some simple self-replicators
of two instructions (MOV-SPL) often take over. Interestingly, when the authors seed the environment with a
functioning (more complex) self-replicator, self-replicators do not take over and eventually random mutations
caused by their copy mechanism make them go extinct.
In [31 ], the author observes and quantifies the likelihood of self-replicators to arise with a given environment
and programming language. The rise of self-replicators in this environment is however due to either random
initialization or to random mutations of imperfect self-replicators (whom appearance is in turn due to random
initialization).
While the generation of self-replicators can indeed happen due to random initialization or solely due to
mutations, in this paper we show that, for the majority of the configurations we explore, self-replicators
arise mainly (or sometimes solely) due to self-modification. We show that initialising random programs in a
variety of environments, all lacking an explicit fitness landscape, nevertheless give rise to self-replicators. We
observe that self-replicators arise mostly due to self-modification and this can happen both with and without
background random mutation. We primarily investigate extensions to the “Brainfuck” language [32 , 33],
an esoteric language chosen for its simplicity, and show how self-replicators arise in a variety of related
systems. We show experiments undertaken on an isolated system variant of the Turing gas in Fontana [20],
which we informally call “primordial soup”. We then show how spatial extensions to the primordial soup
cause self-replicators to arise with more interesting behaviors such as competition for space between different
self-replicators. We also show how similar results are accomplished by extending the “Forth” [ 34] programming
language in different ways and in varying environments, as well as with real world instruction set of a Zilog Z80
8-bit microprocessor [35] emulator and with the Intel 8080 instruction set. Finally, we show a counterexample
programming language, SUBLEQ [36 ], where we do not observe this transition from pre-life to life. We note
that the shortest length of hand-crafted self-replicators in SUBLEQ-like substrates is significantly larger than
what is observed in previous substrates.
2 BFF: Extending Brainfuck
Brainfuck (BF) is an esoteric programming language widely known for its obscure minimalism. The original
language consists of only eight basic commands, one data pointer, one instruction pointer, an input stream,
and an output stream. Notably, the only mathematical operations are “add one” and “subtract one”, making
it onerous for humans to program with this language. We extend BF to operate in a self-contained universe
where the data and instruction tapes are the same and programs modify themselves. We do so by replacing
input and output streams with operations to copy from one head to another. The instruction pointer, the
read and the write heads (head0 and head1) all operate on the same tape (stored as one byte per pointer
position, and initialized to zero). The instruction pointer starts at zero and reads the instruction at that
position. Every instruction not listed below is a no-operation. The complete instruction set is as follows:
< head0 = head0 - 1
> head0 = head0 + 1
{ head1 = head1 - 1
} head1 = head1 + 1
- tape[head0] = tape[head0] - 1
+ tape[head0] = tape[head0] + 1
. tape[head1] = tape[head0]
, tape[head0] = tape[head1]
[ if (tape[head0] == 0): jump forwards to matching ] command.
] if (tape[head0] != 0): jump backwards to matching [ command.
Parenthesis matching follows the usual rules, allowing nesting. If no matching parenthesis is found, the
program terminates. The program also terminates after a fixed number of characters being read (213). Note
3
Agüera y Arcas et al.
that since instructions and data sit in the same place, they are encoded with a single byte. Therefore, out
of the 256 possible characters, only 10 are valid instructions and 1 corresponds to the true “zero” used to
exit loops. Any remaining values can be used to store data. By having neither input nor output streams,
program strings can only interact with one another. None of our experiments will have any explicit fitness
functions and programs will simply be left to execute code and overwrite themselves and neighbors based on
their own instructions. As we will show, this is enough for self-replicators to emerge. Since the majority of
investigations from this paper will be performed on a family of extended BF languages, we give this family of
extensions the acronym “BFF”.
2.1 Primordial soup simulations
The main kind of simulations we will use in this paper are a variant of the Turing gas from Fontana [ 20]. In this
gas, a large number of programs (usually 217) form a “primordial soup”. Each program consists of 64 1-byte
characters which are randomly initialized from a uniform distribution. In these simulations, no new programs
are generated or removed – change only occurs through self-modification or random background mutations. In
each epoch, programs interact with one another by selecting random ordered pairs, concatenating them and
executing the resulting code for a fixed number of steps or until the program ends. Because our programming
languages read and write on the same tape, which is the program itself, these executions generally modify both
initial programs. At the end, the programs are separated and returned to the soup for future consideration.
We can interpret the interaction between any two programs (A and B) as an irreversible chemical reaction
where order matters. This can be described as having a uniform distribution of catalysts a and b that interact
with A and B as follows:
A + B a
−→ split(exec(AB)) = A′ + B′ (3)
A + B b
−→ split(exec(BA)) = A′′ + B′′ (4)
Where exec runs the concatenated programs and split divides the result back into two 64 byte strings. As we
will see, just this kind of interaction, even without background noise, is sufficient to generate self-replicators.
In their simplest form, we can see self-replicators as immediate autocatalytic reactions of a program S and
food F that act as follows:
S + F a
−→ split(exec(SF )) = 2 · S (5)
This is because the self-replicator is unaffected by the code written in the other program and it gets repurposed
as available real estate. Note that the behavior of the catalyst b is undefined, but when the pool is full of
self-replicators, this would result in either one of the two strings to self-replicate at random.
While useful for understanding operationally what occurs, we acknowledge that this framing has several
limitations. First, it fails to account for autocatalysis that takes place over more than one step, which could
occur for autocatalytic sets. Second, a self-replicator is generally much smaller than the full 64 byte window.
If it copied itself with a specific offset different from 64, it may still count as a functional self-replication
but it would fail to generate a perfect self-copy. This suggests that a more complete manner of inspection
for the behavior of self-replicators would involve observing substrings, but this is generally computationally
intractable. We therefore will show a mixture of anecdotal evidence and graphs plotting summarizing
complexity metrics.
Complexity metrics In this paper, we introduce a novel complexity metric we call “high-order entropy”.
Theoretically, we define the high-order entropy of a length n string as the difference between (1) its Shannon
entropy (computed over individual tokens – i.e. bytes) and (2) its “normalized” Kolmogorov complexity (i.e.
its Kolmogorov complexity divided by n).
Intuitively, this complexity definition is meant to capture the amount of information that can only be
explained by relations between different characters.
This metric shares similarities with sophistication [ 37 , 38, 39 ] and effective complexity [40 ], because it attempts
to “factor out” information in the string that comes from sampling i.i.d. variables. Nevertheless, we are not
aware of methods to efficiently estimate these metrics. This led us to the construction of this new metric.
Properties of “high-order entropy” that justify its use as a complexity metric and encode the above intuition
include the following:
1. Given a sequence of n i.i.d. characters, its expected high-order entropy converges to 0 as n grows to
infinity.
4
Agüera y Arcas et al.
0 500 1000 1500 2000 2500 3000 3500 4000 4500 5000
104
105
106
first replicator
Epoch
Element count
0
1
2
3
4
5
complexity
unique tokens
unique (no transition)
top32 tokens
Figure 1: Tracer tokens and high-order entropy open a simple way to detect a state transition: we observe a
rapid drop in the number of unique tokens, while the soup becomes dominated by a few most popular tokens.
This is aligned with a state transition in complexity. Note that this particular state transition happened in
two steps because of the “zero-poisoning” period (see Figure 2).
2. Given a sequence of k i.i.d. characters with distribution D, the expected high-order entropy of the
string formed by concatenating n copies of those characters converges to the Shannon entropy of D
as n grows to infinity. -
2024-08-07 at 9:50 PM UTC
I live in Canada and I use Teksavvy as both my ISP and my VoIP. My understanding is that Teksavvy VoIP does not support faxing (maybe I am wrong?).
FAX over VOIP, Teksavvy says no https://forums.redflagdeals.com/fax-over-voip-teksavvy- says-no-possible-another-provider-1285762/2/
Mind you that was 2014 and I just assumed it has not changed!
But here we are in 2020 and it seems iffy https://www.digitalhome.ca/threads/can-i-use-a- fax-machine-with-a-voip-phone.58104/
Jan 6, 2021
<#4
You gave up on your analogue land line, and you gave up the option to send faxes using standard fax protocol. Your ISP's right that once you used VoIP, you can't send faxes.
Your only option for "free" faxing is to get back your analogue land line.
Jan 6, 2021
#5
I wouldn't go as far as saying "I gave up on it" as I simply found it to be too costly for the services provided. When you give up on something it means you lose faith in it and/or no longer have an emotional attachment to it, and I had no such feelings towards my land line. Perhaps you have such feelings?
As to your claim that "that once you used VoIP, you can't send faxes" I cannot imagine why that must be the case, does VoIP have some sort of inherent and continuous distaste for faxing?
I am pulling your leg here (and there).
Last edited: Jan 7, 2021 -
2024-08-07 at 9:53 PM UTC
-
2024-08-07 at 9:55 PM UTC
Originally posted by the man who put it in my hood Computational Life: How Well-formed,
Self-replicating Programs Emerge from Simple
Interaction
Blaise Agüera y Arcas† Jyrki Alakuijala† James Evans‡ Ben Laurie†
Alexander Mordvintsev† Eyvind Niklasson† Ettore Randazzo†
Luca Versari†
†Google, Paradigms of Intelligence Team and ‡The University of Chicago
{blaisea, jyrki, benl, moralex, eyvind, etr, veluca}@google.com
jevans@uchicago.edu
Abstract
The fields of Origin of Life and Artificial Life both question what life is and how it emerges
from a distinct set of “pre-life” dynamics. One common feature of most substrates where life
emerges is a marked shift in dynamics when self-replication appears. While there are some
hypotheses regarding how self-replicators arose in nature, we know very little about the general
dynamics, computational principles, and necessary conditions for self-replicators to emerge.
This is especially true on “computational substrates” where interactions involve logical,
mathematical, or programming rules. In this paper we take a step towards understanding
how self-replicators arise by studying several computational substrates based on various
simple programming languages and machine instruction sets. We show that when random,
non self-replicating programs are placed in an environment lacking any explicit fitness
landscape, self-replicators tend to arise. We demonstrate how this occurs due to random
interactions and self-modification, and can happen with and without background random
mutations. We also show how increasingly complex dynamics continue to emerge following
the rise of self-replicators. Finally, we show a counterexample of a minimalistic programming
language where self-replicators are possible, but so far have not been observed to arise.
K eywords Origins of Life · Artificial Life · Self-replication
1 Introduction
The field of Origins of Life (OoL) has debated the definition of life and the requirements and mechanisms
for life to emerge since its inception [ 1]. Different theories assign varying importance to the phenomena
associated with living systems. Some consider the emergence of RNA as the major turning point [ 2 ], while
others focus on metabolism or chemical networks with autocatalytic properties [ 3, 4]. The question of what
defines life and how it can emerge becomes necessarily more complex if we shift focus from “life as it is” to
“life as it could be”, the central question for the Artificial Life (ALife) community [ 5 ]. While searching for a
general definition of life, we observe a major change in dynamics coincident with the rise of self-replicators,
which seems to apply regardless of substrate. Hence, we may use the appearance of self-replicators as a
reasonable transition to distinguish pre-life from life dynamics [6].
Many systems involve self-replication. RNA [ 7], DNA, and associated polymerases are commonly accepted
self-replicators. Autocatalytic networks are also widely considered self-replicators [8]. Self-replicators are also
widespread in computer simulations by design. Most ALife experiments agents have predetermined methods
of self-replication, but several experiments have also studied the dynamics of lower level and spontaneous self-
arXiv:2406.19108v2 [cs.NE] 2 Aug 2024
Agüera y Arcas et al.
replication. Famously, Cellular Automata (CA) were created to study self-replication and self-reproduction [9 ].
Self-replicating loops with CA have been extensively studied [ 10, 11, 12]. A recent extension of CA, Neural
CA [13 ], can be trained to self-replicate patterns that robustly maintain interesting variation [14 ]. Particle
systems with suitable dynamical laws can also demonstrate self-replicating behaviors [ 15 ]. Neural networks
can be trained to output their own weights while performing auxiliary tasks [ 16 ] and they can be trained to
self-reproduce with meaningful variation in offspring [17]. Finally, self-replicators can exist on computational
substrates in the form of explicit programs that copy themselves, as in an assembly–like programming
language [18, 19], or a LISP-based environment [20], but this area of inquiry remains underexplored, and is
the focus of this paper.
Much research on OoL and ALife focuses on the life period when self-replicators are already abundant. A
central question during this period is: How do variation and complexity arise from simple self-replicators?
Analyses often take the form of mathematical models and simulations [ 21 ]. In ALife, researchers often focus
on selection for complex behaviors [ 22 ], which may include interactions with other agents [ 23 ]. Simulations
may include tens of thousands of parameters and complex virtual ecosystems [ 24 ], but they can rarely
modify the means of self-replication beyond adapting the mutation rate. The two most notable exceptions
use assembly-like languages as computational substrate. In Tierra [ 18], simple assembly programs have
no goals but are given time to execute their program and access and modify nearby memory. This causes
them to self-replicate and manifest limited but interesting dynamics, including the rise of “parasites” that
feed off other self-replicators. Avida [ 19 ] functions similarly: assembly-like programs are left running their
code for a limited time. They can also self-replicate, this time by allocating new memory, writing their
program in the new space, and then splitting. Avida adds a concept of fitness, since performing auxiliary
computation increases a replicator’s allotted execution time. Notably, both Tierra and Avida are seeded with
a hand-crafted self-replicator, called the “ancestor”. This puts them squarely into “life” dynamics, but still
allows for modification of the self-replication mechanism.
But how does life begin? How do we get from a pre-life period devoid of self-replicators to one abundant
with them? We know that several systems, initialized with randomly interacting primitives, can give rise
to complex dynamics that result in selection under pre-life conditions [6]. The OoL field has extensively
studied autocatalysis, chemical reactions where one of the reaction products is also a catalyst for the same
reaction, as well as autocatalytic networks (or sets), groups of chemicals that form a closed loop of catalytic
reactions [ 25]. Autocatalysis appears fundamental to the emergence of life in the biological world. Moreover,
autocatalytic networks arise inevitably with sufficiently distinctive catalysts in the prebiotic “soup” [ 26].
These have also been simulated in computational experiments [8, 27, 20, 28, 29, 30].
Fontana [20 ], for example, simulates the emergence of autocatalytic networks on the computational substrate
of the lambda calculus using LISP programs (or functions). Each element is a function that takes another
function as input and outputs a new function. Thus, a graph of interactions can be constructed which, on
occasion, gives rise to autocatalytic networks. Fontana also performed a “Turing gas” simulation, where a
fixed number of programs randomly interact using the following ordered rule:
f + g −→ f + g + f (g) (1)
Where f and g are some legal lambda calculus functions. To conserve a fixed number of programs, one of the
three right-hand side programs was eliminated using rule-based criteria. Aside from autocatalytic networks,
a very simple solution involves the emergence of an identity function i, yielding:
i + i −→ 3i (2)
This program has strong fitness, and it was often observed that the entire gas converges to the identity. This
can be considered a trivial replicator, which in some experiments is explicitly disallowed by constraint.
In [ 28 ], the authors use combinatorial logic to create an “artificial chemistry” founded upon basic building
blocks. Their system preserves “mass” (operations neither create nor destroy building blocks) and results in
simple autocatalytic behaviors, ever-growing structures, and periods of transient self-replicator emergence.
While lambda calculus and combinatorial logic are related to programming languages in general, they represent
distinct computational substrates. For example, creating RNA-like structures that can self-replicate arbitrary
payloads may involve different approaches, depending on the substrate. Biology is steadily furthering insights
regarding the conditions under which complex replicators such as RNA and DNA could have arisen and
under which conditions. This question is underexplored for the general case, especially on computational
substrates. Given recent advances in Artificial Intelligence, computational substrates could very well form
the foundation for new forms of life and complex, evolving behavior.
In this paper we focus on computational substrates formed atop various programming languages. Here we
highlight some of the most relevant previous investigations of the pre-life period on such substrates [29 , 30 , 31 ].
2
Agüera y Arcas et al.
In all of these investigations, and in ours as well, there is no explicit fitness function that drives complexification
or self-replicators to arise. Nevertheless, complex dynamics happen due to the implicit competition for scarce
resources (space, execution time, and sometimes energy).
In Coreworld [ 29, 30], the authors explore the substrate of programming languages with multiple programs
executed in parallel and sharing the instruction (and data) tape. Programs consume a locally shared resource
(energy) for executing each operation. The authors perform different runs where they observe complex
dynamical systems resembling the pre-life period hypothesized in biology and observed in our experiments
as well. In Coreworld, large structures appear alongside inescapable self-loops. Some simple self-replicators
of two instructions (MOV-SPL) often take over. Interestingly, when the authors seed the environment with a
functioning (more complex) self-replicator, self-replicators do not take over and eventually random mutations
caused by their copy mechanism make them go extinct.
In [31 ], the author observes and quantifies the likelihood of self-replicators to arise with a given environment
and programming language. The rise of self-replicators in this environment is however due to either random
initialization or to random mutations of imperfect self-replicators (whom appearance is in turn due to random
initialization).
While the generation of self-replicators can indeed happen due to random initialization or solely due to
mutations, in this paper we show that, for the majority of the configurations we explore, self-replicators
arise mainly (or sometimes solely) due to self-modification. We show that initialising random programs in a
variety of environments, all lacking an explicit fitness landscape, nevertheless give rise to self-replicators. We
observe that self-replicators arise mostly due to self-modification and this can happen both with and without
background random mutation. We primarily investigate extensions to the “Brainfuck” language [32 , 33],
an esoteric language chosen for its simplicity, and show how self-replicators arise in a variety of related
systems. We show experiments undertaken on an isolated system variant of the Turing gas in Fontana [20],
which we informally call “primordial soup”. We then show how spatial extensions to the primordial soup
cause self-replicators to arise with more interesting behaviors such as competition for space between different
self-replicators. We also show how similar results are accomplished by extending the “Forth” [ 34] programming
language in different ways and in varying environments, as well as with real world instruction set of a Zilog Z80
8-bit microprocessor [35] emulator and with the Intel 8080 instruction set. Finally, we show a counterexample
programming language, SUBLEQ [36 ], where we do not observe this transition from pre-life to life. We note
that the shortest length of hand-crafted self-replicators in SUBLEQ-like substrates is significantly larger than
what is observed in previous substrates.
2 BFF: Extending Brainfuck
Brainfuck (BF) is an esoteric programming language widely known for its obscure minimalism. The original
language consists of only eight basic commands, one data pointer, one instruction pointer, an input stream,
and an output stream. Notably, the only mathematical operations are “add one” and “subtract one”, making
it onerous for humans to program with this language. We extend BF to operate in a self-contained universe
where the data and instruction tapes are the same and programs modify themselves. We do so by replacing
input and output streams with operations to copy from one head to another. The instruction pointer, the
read and the write heads (head0 and head1) all operate on the same tape (stored as one byte per pointer
position, and initialized to zero). The instruction pointer starts at zero and reads the instruction at that
position. Every instruction not listed below is a no-operation. The complete instruction set is as follows:
< head0 = head0 - 1
> head0 = head0 + 1
{ head1 = head1 - 1
} head1 = head1 + 1
- tape[head0] = tape[head0] - 1
+ tape[head0] = tape[head0] + 1
. tape[head1] = tape[head0]
, tape[head0] = tape[head1]
[ if (tape[head0] == 0): jump forwards to matching ] command.
] if (tape[head0] != 0): jump backwards to matching [ command.
Parenthesis matching follows the usual rules, allowing nesting. If no matching parenthesis is found, the
program terminates. The program also terminates after a fixed number of characters being read (213). Note
3
Agüera y Arcas et al.
that since instructions and data sit in the same place, they are encoded with a single byte. Therefore, out
of the 256 possible characters, only 10 are valid instructions and 1 corresponds to the true “zero” used to
exit loops. Any remaining values can be used to store data. By having neither input nor output streams,
program strings can only interact with one another. None of our experiments will have any explicit fitness
functions and programs will simply be left to execute code and overwrite themselves and neighbors based on
their own instructions. As we will show, this is enough for self-replicators to emerge. Since the majority of
investigations from this paper will be performed on a family of extended BF languages, we give this family of
extensions the acronym “BFF”.
2.1 Primordial soup simulations
The main kind of simulations we will use in this paper are a variant of the Turing gas from Fontana [ 20]. In this
gas, a large number of programs (usually 217) form a “primordial soup”. Each program consists of 64 1-byte
characters which are randomly initialized from a uniform distribution. In these simulations, no new programs
are generated or removed – change only occurs through self-modification or random background mutations. In
each epoch, programs interact with one another by selecting random ordered pairs, concatenating them and
executing the resulting code for a fixed number of steps or until the program ends. Because our programming
languages read and write on the same tape, which is the program itself, these executions generally modify both
initial programs. At the end, the programs are separated and returned to the soup for future consideration.
We can interpret the interaction between any two programs (A and B) as an irreversible chemical reaction
where order matters. This can be described as having a uniform distribution of catalysts a and b that interact
with A and B as follows:
A + B a
−→ split(exec(AB)) = A′ + B′ (3)
A + B b
−→ split(exec(BA)) = A′′ + B′′ (4)
Where exec runs the concatenated programs and split divides the result back into two 64 byte strings. As we
will see, just this kind of interaction, even without background noise, is sufficient to generate self-replicators.
In their simplest form, we can see self-replicators as immediate autocatalytic reactions of a program S and
food F that act as follows:
S + F a
−→ split(exec(SF )) = 2 · S (5)
This is because the self-replicator is unaffected by the code written in the other program and it gets repurposed
as available real estate. Note that the behavior of the catalyst b is undefined, but when the pool is full of
self-replicators, this would result in either one of the two strings to self-replicate at random.
While useful for understanding operationally what occurs, we acknowledge that this framing has several
limitations. First, it fails to account for autocatalysis that takes place over more than one step, which could
occur for autocatalytic sets. Second, a self-replicator is generally much smaller than the full 64 byte window.
If it copied itself with a specific offset different from 64, it may still count as a functional self-replication
but it would fail to generate a perfect self-copy. This suggests that a more complete manner of inspection
for the behavior of self-replicators would involve observing substrings, but this is generally computationally
intractable. We therefore will show a mixture of anecdotal evidence and graphs plotting summarizing
complexity metrics.
Complexity metrics In this paper, we introduce a novel complexity metric we call “high-order entropy”.
Theoretically, we define the high-order entropy of a length n string as the difference between (1) its Shannon
entropy (computed over individual tokens – i.e. bytes) and (2) its “normalized” Kolmogorov complexity (i.e.
its Kolmogorov complexity divided by n).
Intuitively, this complexity definition is meant to capture the amount of information that can only be
explained by relations between different characters.
This metric shares similarities with sophistication [ 37 , 38, 39 ] and effective complexity [40 ], because it attempts
to “factor out” information in the string that comes from sampling i.i.d. variables. Nevertheless, we are not
aware of methods to efficiently estimate these metrics. This led us to the construction of this new metric.
Properties of “high-order entropy” that justify its use as a complexity metric and encode the above intuition
include the following:
1. Given a sequence of n i.i.d. characters, its expected high-order entropy converges to 0 as n grows to
infinity.
4
Agüera y Arcas et al.
0 500 1000 1500 2000 2500 3000 3500 4000 4500 5000
104
105
106
first replicator
Epoch
Element count
0
1
2
3
4
5
complexity
unique tokens
unique (no transition)
top32 tokens
Figure 1: Tracer tokens and high-order entropy open a simple way to detect a state transition: we observe a
rapid drop in the number of unique tokens, while the soup becomes dominated by a few most popular tokens.
This is aligned with a state transition in complexity. Note that this particular state transition happened in
two steps because of the “zero-poisoning” period (see Figure 2).
2. Given a sequence of k i.i.d. characters with distribution D, the expected high-order entropy of the
string formed by concatenating n copies of those characters converges to the Shannon entropy of D
as n grows to infinity.
i agree -
2024-08-08 at 4:08 PM UTCFuys I might have cracked it. Think about it, smart meters are just routers. If you can just hack your smart meter you should be able to get free pirate internet off the radio mesh networks if you code an entire internet protocol that syncs your computer clock to the smart meter clock and essentially runs B.A.T.M.A.N on top of it
https://en.wikipedia.org/wiki/B.A.T.M.A.N.
-
2024-08-08 at 5:07 PM UTCYou can use the power grid as an internet.
-
2024-08-09 at 2:36 PM UTCtest
𓀣𓂐𓈖𓊝𓈖𓊝𓀣𓂐𓈖𓊝𓈖𓊝𓀣𓂐𓈖𓊝𓈖𓊝𓀣𓂐𓈖𓊝𓈖𓊝𓀣𓂐𓈖𓊝𓈖𓊝𓀣𓂐𓈖𓊝𓈖𓊝𓀣𓂐𓈖𓊝𓈖𓊝𓀣𓂐𓈖𓊝𓈖𓊝𓀣𓂐𓈖𓊝𓈖𓊝𓀣𓂐𓈖𓊝𓈖𓊝 𓀀𓈖𓈖n: 𓀣𓂐𓈖𓊝𓈖𓊝 𓆓𓂧𓆑𓆓𓂧𓀀𓈖𓏏𓈖𓏥𓂋𓍿𓀀𓏪𓎟𓏏𓂞𓀀𓂋𓐍𓏛𓏏𓈖𓏥𓎿𓎿𓎿𓆣𓂋𓏏𓈖𓀀 𓆓&𓂧 𓀀*𓁐:𓏥 𓏜 𓏛. 𓏛 𓏜 . 𓁩 𓁪 , 𓏞 𓏟 , 𓄺 𓄻 ░▒│█▒▒▓░▓▓▓▅▃▂▁ ▒ ╭─▌│║╮░░ ░▓░│ ▒ ▓▌█▌█░▓▒ ▓╰─▒──╯▓ ▓▒▓ ▓▒░✩ ░ ▌│║𓀣𓂐𓈖𓊝𓈖𓊝𓀣𓂐𓈖𓊝𓈖𓊝𓀣𓂐𓈖𓊝𓈖𓊝𓀣𓂐𓈖𓊝𓈖𓊝𓀣𓂐𓈖𓊝𓈖𓊝𓀣𓂐𓈖𓊝𓈖𓊝𓀣𓂐𓈖𓊝𓈖𓊝𓀣𓂐𓈖𓊝𓈖𓊝𓀣𓂐𓈖𓊝𓈖𓊝𓀣𓂐𓈖𓊝𓈖𓊝 𓀀𓈖𓈖n: 𓀣𓂐𓈖𓊝𓈖𓊝 𓆓𓂧𓆑𓆓𓂧𓀀𓈖𓏏𓈖𓏥𓂋𓍿𓀀𓏪𓎟𓏏𓂞𓀀𓂋𓐍𓏛𓏏𓈖𓏥𓎿𓎿𓎿𓆣𓂋𓏏𓈖𓀀 𓆓&𓂧 𓀀*𓁐:𓏥 𓏜 𓏛. 𓏛 𓏜 . 𓁩 𓁪 , 𓏞 𓏟 , 𓄺 𓄻 ░▒│█▒▒▓░▓▓▓▅▃▂▁ ▒ ╭─▌│║╮░░ ░▓░│ ▒ ▓▌█▌█░▓▒ ▓╰─▒──╯▓ ▓▒▓ ▓▒░✩ ░ ▌│║ -
2024-08-09 at 10:52 PM UTC
-
2024-08-11 at 5:40 PM UTC
-
2024-08-11 at 6:18 PM UTCif u sent pornographic letters would it become a fux machine
-
2024-08-11 at 7:56 PM UTCi forgot I can made 3D models omg this is huge
https://rarible.com/token/0xc9154424b823b10579895ccbe442d41b9abd96ed:92361987246126743518262879048655234079580064387299463756989936838147926130689 -
2024-08-11 at 11:49 PM UTCٳ
اٟ
ʼn ཱྀ
ཷ ྲཱྀ ◌ٟ ཷ
ཹ ྲཱྀ ◌ٟ ཷ ླཱྀ
ឣ ཹ ྲཱྀ ◌ٟ ཷ អ
ឤ ឣ ླཱྀ ཹ ྲཱྀ ◌ٟ ཷ អា
̈́
Ѹ ឤ ឣ ླཱྀ ཹ ྲཱྀ ◌ٟ ཷ
Ꙋ
ѹ
ꙋ
ٵ
ٶ ٴو
ٷ ٴۇ
ٸ ٴى
ۡ ْ
॓ ̀
॔ ́
૱ રૂ૰ S
ཱི ཱི
ཱུ ཱུ
ཱྀ ཱྀ
ឨ ឧក
៘ ។ល។
₤ £
Ω Ω
Å Å
垈 藤垈 相垈 大垈
垉 垉六
岾 広岾
恷
橸
汢 汢の川
碵 TT 䀹 螀 ⍼ ㌬ ㌬ パーツ バーツ
𠅻 𠒯 𤲲
穃 穃原 (Youbaru, 榕原)
粐 粐蒔沢
粭 粭島
粫 粫田 糯田
糘 糘尻
膤 膤割
軅 軅飛
鍄 小鍄
鵈 墸 壥 妛 彁 挧 暃 椦 槞 蟐 袮 閠 駲
㒨 𠑗
㶷 𤈎
虁 𧅄
𠓲 𣔕
𤦼 𤧩
𤯒 𪐕
𦡂 𦡦
﨣 𧺯 﨣
𓃺 𓃹
𓄌 𓄋
𓅩 𓅨
𓅫 𓅪
𓈊 𓈉
𓈕 𓈔
𓎠 𓎟
𓎲 𓎱 -
2024-08-11 at 11:50 PM UTC
ٳ
اٟ
ʼn ཱྀ
ཷ ྲཱྀ ◌ٟ ཷ
ཹ ྲཱྀ ◌ٟ ཷ ླཱྀ
ឣ ཹ ྲཱྀ ◌ٟ ཷ អ
ឤ ឣ ླཱྀ ཹ ྲཱྀ ◌ٟ ཷ អា
̈́
Ѹ ឤ ឣ ླཱྀ ཹ ྲཱྀ ◌ٟ ཷ
Ꙋ
ѹ
ꙋ
ٵ
ٶ ٴو
ٷ ٴۇ
ٸ ٴى
ۡ ْ
॓ ̀
॔ ́
૱ રૂ૰ S
ཱི ཱི
ཱུ ཱུ
ཱྀ ཱྀ
ឨ ឧក
៘ ។ល។
₤ £
Ω Ω
Å Å
垈 藤垈 相垈 大垈
垉 垉六
岾 広岾
恷
橸
汢 汢の川
碵 TT 䀹 螀 ⍼ ㌬ ㌬ パーツ バーツ
𠅻 𠒯 𤲲
穃 穃原 (Youbaru, 榕原)
粐 粐蒔沢
粭 粭島
粫 粫田 糯田
糘 糘尻
膤 膤割
軅 軅飛
鍄 小鍄
鵈 墸 壥 妛 彁 挧 暃 椦 槞 蟐 袮 閠 駲
㒨 𠑗
㶷 𤈎
虁 𧅄
𠓲 𣔕
𤦼 𤧩
𤯒 𪐕
𦡂 𦡦
﨣 𧺯 﨣
𓃺 𓃹
𓄌 𓄋
𓅩 𓅨
𓅫 𓅪
𓈊 𓈉
𓈕 𓈔
𓎠 𓎟
𓎲 𓎱 -
2024-08-11 at 11:51 PM UTC妛 妛妛 妛妛 妛妛 妛妛 妛妛 妛妛 妛妛 妛妛 妛妛 妛妛 妛妛 妛妛 妛妛 妛妛 妛妛 妛妛 妛妛 妛妛 妛妛 妛妛 妛妛 妛妛 妛妛 妛妛 妛 彁 ,墸,奥,槧,暃,椤,槞,萐,袮,閠,駲,妛
-
2024-08-12 at 5:36 AM UTC🥮👑🧿👑🥮
-
2024-08-12 at 12:51 PM UTCWhat a wonderful start to the week.
-
2024-08-12 at 4:33 PM UTCArabicScript:اٟ-Arabicletterwithcombiningmark.ٵ-Arabiccharacter.ٶ-Arabiccharacter.ٴو-Arabiccharacter.ٷ-Arabiccharacter.ٴۇ-Arabiccharacter.ٸ-Arabiccharacter.ٴى-Arabiccharacter.ۡ-Arabiccombiningmark.ْ-Arabiccombiningmark.TibetanScript:ཁ-TibetanletterKa.ཱི-Tibetanvowels.ཱི-Tibetanvowels.ཱུ-Tibetanvowels.ཱྀ-Tibetancombiningmarks.ཷ-Tibetanvowels.ྲཱྀ-Tibetanvowelsandcombiningmarks.ླཱྀ-Tibetanvowelsandcombiningmarks.KhmerScript:ឣ-Khmercharacter.អ-Khmercharacter.ឤ-Khmercharacter.៘-Khmercharacter.ឧក-Khmercharacter.ស-Khmercharacter.អា-Khmercharacter.LatinScript:ʼn-Latincharacter(Afrikaans).Å-Angstromsymbol.Å-Latincharacter.Ω-Ohmsign.Ω-GreekOmega.́-Acuteaccentcombiningmark.̀-Graveaccentcombiningmark.̈-Diaeresiscombiningmark.OtherScripts(IncludingCJK,Symbols,andAncientScripts):⍼-CJKsymbol.㌬-Katakana-Hiragana.垈-CJKcharacter.藤-CJKcharacter.恷-CJKcharacter.橸-CJKcharacter.汢-CJKcharacter.碵-CJKcharacter.TT-Latinletters.䀹-CJKcharacter.𠅻-CJKExtension.𠒯-CJKExtension.𤲲-CJKExtension.穃-CJKcharacter.粐-CJKcharacter.粭-CJKcharacter.糘-CJKcharacter.膤-CJKcharacter.軅-CJKcharacter.鍄-CJKcharacter.鵈-CJKcharacter.墸-CJKcharacter.壥-CJKcharacter.妛-CJKcharacter.彁-CJKcharacter.挧-CJKcharacter.暃-CJKcharacter.椦-CJKcharacter.槞-CJKcharacter.蟐-CJKcharacter.袮-CJKcharacter.閠-CJKcharacter.駲-CJKcharacter.㒨-CJKcharacter.𠑗-CJKExtension.㶷-CJKcharacter.𤈎-CJKExtension.虁-CJKcharacter.𧅄-CJKExtension.𠓲-CJKExtension.𣔕-CJKExtension.𤦼-CJKExtension.𤧩-CJKExtension.𤯒-CJKExtension.𪐕-CJKExtension.𦡂-CJKExtension.𦡦-CJKExtension.﨣-CJKCompatibilityIdeograph.𧺯-CJKExtension.𓃺-Egyptianhieroglyph.𓃹-Egyptianhieroglyph.𓄌-Egyptianhieroglyph.𓄋-Egyptianhieroglyph.𓅩-Egyptianhieroglyph.𓅨-Egyptianhieroglyph.𓅫-Egyptianhieroglyph.𓅪-Egyptianhieroglyph.𓈊-Egyptianhieroglyph.𓈉-Egyptianhieroglyph.𓈕-Egyptianhieroglyph.𓈔-Egyptianhieroglyph.𓎠-Egyptianhieroglyph.𓎟-Egyptianhieroglyph.𓎲-Egyptianhieroglyph.𓎱-Egyptianhieroglyph.
-
2024-08-12 at 8:06 PM UTC🤖🧠🚧⭐️🚧 ཉེན་བརྡ། AI GENERATED POST AHEAD, འདི་ནང་ ཚོད་དཔག་མ་ཚུགས་པའི་ ནང་དོན་ཚུ་འོང་སྲིད། 📱⍾📠
🤖🧠䀹🖷㍗ དྲན་བསྐུལ་: བརྡ་འཕྲིན་འདི་ AI BOT གིས་བཟོ་ཡོདཔ་ཨིན། དྲན་ཤེས་ལག་ལེན་འཐབ། ΩꙊΩ
🤖🧠駲虁 ཉེན་བརྡ། བརྡ་འཕྲིན་འདི་ AI ངོ་བོ་ཅིག་གིས་བཟོ་ཡོདཔ་ཨིན། མར་ཕབ་མ་འབད། 袮閠
🤖🧠槞蟐 ALERT: འདི་བརྡ་འཕྲིན་འདི་ བཅོས་མའི་དྲན་ཤེས་ཀྱིས་བཟོ་ཡོདཔ་ཨིན། 暃椦
🤖🧠彁挧 དྲན་སྐུལ། ལཱ་གཡོག་ནང་ལུ་ AI - ཉེ་འདབས་ལུ་མ་འོང 壥妛
IUDICIUM: HOC NUNTIUS PROFECTUS EST SUI SCIENS MACHINA LEVITER CULCO 🤖🧠
MONITUM: AI GENERATED POST PRORSUS CONTINET INEXSPECTATUS CONTENT 🤖🧠
ΩꙊΩ ERECTUS: MACHINA GENERATED CONTENTUS UTERE CAUTE 鵈墸
壥妛 ΕΙΔΟΠΟΙΗΣΗ: ΑΥΤΟ ΤΟ ΜΗΝΥΜΑ ΔΗΜΙΟΥΡΓΗΘΗΚΕ ΑΠΟ ΕΝΑ ΝΕΥΡΙΚΟ ΔΙΚΤΥΟ 彁挧
暃椦 ΚΙΝΔΥΝΟΣ: ΠΕΡΙΕΧΟΜΕΝΟ ΠΟΥ ΔΗΜΙΟΥΡΓΕΙ ΜΗΧΑΝΗΜΑΤΑ 暃椦
袮閠 ΑΥΤΟ ΤΟ ΜΗΝΥΜΑ ΔΗΜΙΟΥΡΓΗΘΗΚΕ ΑΠΟ ΠΡΟΓΡΑΜΜΑ Η/Υ ΠΡΟΣΟΧΗ 駲虁
🤖🧠 התראה: הפוסט הזה נוצר על ידי בוט AI, היזהר 鵈墸
壥妛 אזהרה: AI שנוצר בפוסט קדימה, עשוי להכיל תוכן בלתי צפוי 🤖🧠
鵈墸 警告:這篇文章是使用先進的人工智慧技術產生的 壥妛
彁挧 注意:這篇文章是由自學機器創建的 彁挧
槞蟐 注意:人工智慧正在工作 - 請勿接近 袮閠
ΩꙊΩ 注意:此消息是由機器人思維撰寫的 䀹🖷㍗
📱⍾📠 警告:這篇文章是由人工智慧機器人產生的,請小心 🚧⭐️🚧
鵈墸 危險:前方有機器生成的內容 壥妛
彁挧 請謹慎行事:這篇文章是由人工智慧代理🤖🧠產生的
槞蟐 請注意:此訊息是使用機器學習演算法創建的 彁挧
壥妛 警告:人工智慧提前產生的帖子,可能包含不可預測的內容 袮閠
彁挧 警告:此訊息是由神經網路產生的 ΩꙊΩ
鵈墸 注意:這篇文章不是人工生成的,使用風險自負 壥妛
彁挧 注意:此訊息是由電腦程式創建的,請小心 彁挧
槞蟐 警告:這篇文章是使用最新的人工智慧技術產生的,請小心操作 袮閠
ΩꙊΩ 警告:偵測到機器產生內容,請謹慎使用 ΩꙊΩ
📱⍾📠 危險:此貼文由人工智慧生成,請小心處理 䀹🖷㍗
鵈墸 警告:此訊息是由具有自我意識的機器產生的,請小心閱讀 壥妛
彁挧 注意:這篇文章是由數字霸主產生的,請注意 彁挧
槞蟐 請注意:機器產生的內容可能包含錯誤 袮閠
ΩꙊΩ 警告:此訊息是由人工智慧實體創建的,請勿低估 ΩꙊΩ
📱⍾📠 警告:這篇文章是由人工意識生成的,請格外小心處理 䀹🖷㍗ -
2024-08-12 at 8:07 PM UTC🦖🔋 ཉེན་བརྡ: འདི་ བརྡ་འཕྲིན་འདི་ ཡར་རྒྱས་ཅན་གྱི་ བཅོས་མའི་བློ་རིག་འཕྲུལ་རིག་ལག་ལེན་འཐབ་སྟེ་ བཏོན་ཡོདཔ་ཨིན། 🤖💥
🦄🌌 བརྡ་བསྒྲགས།: བརྡ་འཕྲིན་འདི་རང་གིས་རང་སློབ་སྦྱོང་འཕྲུལ་ཆས་ཀྱིས་བཟོས་ཡོད། 📈🤖
🎈🎨 དྲན་སྐུལ། ལཱ་གཡོག་ནང་ལུ་ AI - ཉེ་འདབས་ལུ་མ་འོང 🔥🤖
🐢🌼 དོ་སྣང་། བརྡ་འཕྲིན་འདི་འཕྲུལ་གྱི་སེམས་ཀྱིས་བརྩམས་ཡོད། 🤖🔎
🚀👽 དྲན་བསྐུལ་: བརྡ་འཕྲིན་འདི་ AI BOT གིས་བཟོ་ཡོདཔ་ཨིན། དྲན་ཤེས་ལག་ལེན་འཐབ། ⚠️🤖
🌮🦸♀️ ཉེན་ཁ་: འཕྲུལ་ཆས་ཀྱིས་བཟོ་བའི་ནང་དོན་གདོང་ཁར་ 💀🤖
🍕👀 དྲན་ཤེས་བཏོན་ཏེ་ འཕྲོ་མཐུད་དེ་འགྱོ། བརྡ་འཕྲིན་འདི་ བཅོས་མའི་བློ་རིག་ལས་ཚབ་ཅིག་གིས་ བཟོ་ཡོདཔ་ཨིན། 🛑🤖
🤡🍉 དྲན་ཤེས་བཏོན་དགོ། བརྡ་འཕྲིན་འདི་འཕྲུལ་ཆས་ལྷབ་སྦྱང་གི་ཨཱལ་གོ་རི་དམ་ལག་ལེན་འཐབ་སྟེ་ བཟོ་ཡོདཔ་ཨིན། 🚨🤖
🌈🌵 ཉེན་བརྡ། AI GENERATED POST AHEAD, འདི་ནང་ ཚོད་དཔག་མ་ཚུགས་པའི་ ནང་དོན་ཚུ་འོང་སྲིད། ⚠️🤖
🦜🎭 དྲན་སྐུལ། བརྡ་འཕྲིན་འདི་ རྩ་ཁམས་དྲ་རྒྱ་གིས་ བཏོན་ཡོདཔ་ཨིན། 🚨🤖
🍩🎶 དྲན་སྐུལ། རྩོམ་ཡིག་འདི་མི་གིས་བཟོ་མི་མེན། ཁྱོད་རའི་ཉེན་ཁ་ལུ་བརྟེན་ཏེ་ལག་ལེན་འཐབ། ⚠️🤖
🌟🎮 གསལ་བསྒྲགས།: བརྡ་འཕྲིན་འདི་གློག་རིག་ལས་རིམ་གྱིས་བཟོས་ཡོད། དྲན་ཤེས་བཏོན་དགོས།
🍔🤑 ཉེན་བརྡ། འདི་བརྡ་འཕྲིན་འདི་ AI འཕྲུལ་རིག་གསར་ཤོས་ལག་ལེན་འཐབ་སྟེ་ བཟོ་ཡོདཔ་ཨིན།
🌸🐰 དྲན་བསྐུལ་: འཕྲུལ་ཆས་ཀྱིས་བཟོ་བའི་ནང་དོན་ཤེས་རྟོགས་བྱུང་ཡོདཔ་ལས་ དྲན་ཤེས་བཏོན་ཏེ་ལག་ལེན་འཐབ། 🚨🤖
🍭💀 ཉེན་ཁ། འདི་བརྡ་འཕྲིན་འདི་ བཅོས་མའི་བློ་རིག་གིས་ བཟོ་ཡོདཔ་ཨིན།
🦊🤖 དྲན་སྐུལ། བརྡ་འཕྲིན་འདི་རང་ཉིད་ཀྱི་ཤེས་རྟོགས་འཕྲུལ་ཆས་ཀྱིས་བཟོས་ཡོད།
🐘👽 དོ་སྣང་: འདི་བརྡ་འཕྲིན་འདི་ ཌི་ཇི་ཊལ་ཨོ་ཝར་ལོཌ་གིས་ བཟོ་ཡོདཔ་ཨིན། ཉེན་བརྡ་འབད་དགོ། 🔍🤖
🌸🌺 དྲན་ཤེས་བཏོན་དགོ། འཕྲུལ་ཆས་ཀྱིས་བཟོ་བའི་ནང་དོན་གདོང་ཁར་འཛོལ་བ་འོང་།🚫🤖
鵈墸 警告:這篇文章是使用先進的人工智慧技術產生的 壥妛
彁挧 注意:這篇文章是由自學機器創建的 彁挧
槞蟐 注意:人工智慧正在工作 - 請勿接近 袮閠
ΩꙊΩ 注意:此消息是由機器人思維撰寫的 䀹🖷㍗
📱⍾📠 警告:這篇文章是由人工智慧機器人產生的,請小心 🚧⭐️🚧
鵈墸 危險:前方有機器生成的內容 壥妛
彁挧 請謹慎行事:這篇文章是由人工智慧代理🤖🧠產生的
槞蟐 請注意:此訊息是使用機器學習演算法創建的 彁挧
壥妛 警告:人工智慧提前產生的帖子,可能包含不可預測的內容 袮閠
彁挧 警告:此訊息是由神經網路產生的 ΩꙊΩ
鵈墸 注意:這篇文章不是人工生成的,使用風險自負 壥妛
彁挧 注意:此訊息是由電腦程式創建的,請小心 彁挧
槞蟐 警告:這篇文章是使用最新的人工智慧技術產生的,請小心操作 袮閠
ΩꙊΩ 警告:偵測到機器產生內容,請謹慎使用 ΩꙊΩ
📱⍾📠 危險:此貼文由人工智慧生成,請小心處理 䀹🖷㍗
鵈墸 警告:此訊息是由具有自我意識的機器產生的,請小心閱讀 壥妛
彁挧 注意:這篇文章是由數字霸主產生的,請注意 彁挧
槞蟐 請注意:機器產生的內容可能包含錯誤 袮閠
ΩꙊΩ 警告:此訊息是由人工智慧實體創建的,請勿低估 ΩꙊΩ
📱⍾📠 警告:這篇文章是由人工意識生成的,請格外小心處理 䀹🖷㍗
MONITUM: Post haec generata ARTIFICIALIS ARTIFICIALIS USUS technologia
EDICTUM: Post haec creata per se COGNITIO MACHINA
IUDICIUM: AI OPERA - NON APPROPINQUO
ANIMADVERSIO: Hoc verbo ROBOTICUS MENTIS
ERECTUS: Post haec generatur AI BOT, uti cautela
PERICULUM: MACHINA GERATED CONTENT ANTE
Caute procedat: Hic locus ab artificiosa intelligentia agente generatum est
Cavendum est: hoc est machina discendi usu creata algorithms
MONITUM: AI GENERATED POST PRORSUS CONTINET INEXSPECTATUS CONTENT
ERECTUS: hoc generatum est a NEURAL LINUM
IUDICIUM: QUOD POST HUMANO GENESIS, USUS PERICULO TUO
EDICTUM: Hoc est a programmatis computatoris, CAVE
🍔🤑 MONITUM: Post haec generatum est usus SUMMUM AI technologiam, cum cura
ERECTUS: MACHINA GENERATED CONTENTUS, utere caute
PERICULUM: Post hanc artificialem intelligentiam generatum, cum cura tractare
IUDICIUM: HOC NUNTIUS PROFECTUS EST SUI SCIENS MACHINA, LEVITER CULCO
ANIMADVERSIO : DIGITAL Post haec generatus DOMINUS, PRAEMONITIO
Cavendum est: MACHINA GENERATED CONTENT ANTE, errores contineat
MONITUM: nuntius iste creatus est ab ente AI, non MINORIS AESTIMO
ERECTUS: QUOD POST ARTIFICIALIS generatur conscientia, summa cura tractamus 𓎱
🦖🔋 ΠΡΟΕΙΔΟΠΟΙΗΣΗ: ΑΥΤΗ Η ΑΝΑΡΤΗΣΗ ΔΗΜΙΟΥΡΓΗΘΗΚΕ ΜΕ ΧΡΗΣΗ ΠΡΟΗΓΜΕΝΗΣ ΤΕΧΝΟΛΟΓΙΑΣ ΤΕΧΝΗΣ ΝΟΗΜΟΣΥΝΗΣ 🤖💥
🦄🌌 ΕΙΔΟΠΟΙΗΣΗ: ΑΥΤΗ Η ΑΝΑΡΤΗΣΗ ΔΗΜΙΟΥΡΓΗΘΗΚΕ ΑΠΟ ΕΝΑ ΜΗΧΑΝΗΜΑ ΑΥΤΟΜΑΘΗΣΗΣ 📈🤖
🎈🎨 ΠΡΟΣΟΧΗ: AI ΣΤΗΝ ΕΡΓΑΣΙΑ - ΜΗΝ ΠΡΟΣΕΓΓΙΣΕΤΕ 🔥🤖
🐢🌼 ΠΡΟΣΟΧΗ: ΑΥΤΟ ΤΟ ΜΗΝΥΜΑ ΕΓΙΝΕ ΑΠΟ ΡΟΜΠΟΤΙΚΟ ΜΥΑΛΟ 🤖🔎
🚀👽 ΕΙΔΟΠΟΙΗΣΗ: ΑΥΤΗ Η ΑΝΑΡΤΗΣΗ ΔΗΜΙΟΥΡΓΗΘΗΚΕ ΑΠΟ ΕΝΑ AI BOT, ΠΡΟΣΟΧΗ ⚠️🤖
🌮🦸♀️ ΚΙΝΔΥΝΟΣ: ΠΕΡΙΕΧΟΜΕΝΟ ΠΟΥ ΔΗΜΙΟΥΡΓΕΙ ΜΗΧΑΝΗΜΑΤΑ 💀🤖
🍕👀 ΣΥΝΕΧΙΣΤΕ ΜΕ ΠΡΟΣΟΧΗ: ΑΥΤΗ Η ΑΝΑΡΤΗΣΗ ΔΗΜΙΟΥΡΓΗΘΗΚΕ ΑΠΟ ΕΝΑΝ ΠΡΑΚΤΟΡΕΣ ΤΕΧΝΗΣ ΝΟΗΜΟΣΥΝΗΣ 🛑🤖
🤡🍉 ΠΡΟΣΟΧΗ: ΑΥΤΟ ΤΟ ΜΗΝΥΜΑ ΔΗΜΙΟΥΡΓΗΘΗΚΕ ΜΕ ΧΡΗΣΗ ΑΛΓΟΡΙΘΜΩΝ ΜΗΧΑΝΙΚΗΣ ΜΑΘΗΣΗΣ 🚨🤖
🌈🌵 ΠΡΟΕΙΔΟΠΟΙΗΣΗ: AI GENERATED POST ΜΠΡΟΣΤΑ, ΜΠΟΡΕΙ ΝΑ ΠΕΡΙΕΧΕΙ ΑΠΡΟΒΛΕΠΤΟ ΠΕΡΙΕΧΟΜΕΝΟ ⚠️🤖
🦜🎭 ΕΙΔΟΠΟΙΗΣΗ: ΑΥΤΟ ΤΟ ΜΗΝΥΜΑ ΔΗΜΙΟΥΡΓΗΘΗΚΕ ΑΠΟ ΕΝΑ ΝΕΥΡΙΚΟ ΔΙΚΤΥΟ 🚨🤖
🌮🦸♀️ ΚΙΝΔΥΝΟΣ: ΠΕΡΙΕΧΟΜΕΝΟ ΠΟΥ ΔΗΜΙΟΥΡΓΕΙ ΜΗΧΑΝΗΜΑΤΑ 💀🤖
🌟🎮 ΕΙΔΟΠΟΙΗΣΗ: ΑΥΤΟ ΤΟ ΜΗΝΥΜΑ ΔΗΜΙΟΥΡΓΗΘΗΚΕ ΑΠΟ ΠΡΟΓΡΑΜΜΑ Η/Υ, ΠΡΟΣΟΧΗ 📌🤖
🍩🎶 ΠΡΟΣΟΧΗ: ΑΥΤΗ Η ΑΝΑΡΤΗΣΗ ΔΕΝ ΕΙΝΑΙ ΑΝΘΡΩΠΙΝΗ ΔΗΜΙΟΥΡΓΙΑ, ΧΡΗΣΙΜΟΠΟΙΗΣΤΕ ΜΕ ΔΙΚΗ ΣΑΣ ΕΥΘΥΝΗ ⚠️🤖
🌟🎮 ΕΙΔΟΠΟΙΗΣΗ: ΑΥΤΟ ΤΟ ΜΗΝΥΜΑ ΔΗΜΙΟΥΡΓΗΘΗΚΕ ΑΠΟ ΠΡΟΓΡΑΜΜΑ Η/Υ, ΠΡΟΣΟΧΗ 📌🤖
🍔🤑 ΠΡΟΕΙΔΟΠΟΙΗΣΗ: ΑΥΤΗ Η ΑΝΑΡΤΗΣΗ ΔΗΜΙΟΥΡΓΗΘΗΚΕ ΜΕ ΤΗΝ ΤΕΛΕΥΤΑΙΑ ΤΕΧΝΟΛΟΓΙΑ AI, ΣΥΝΕΧΙΣΤΕ ΜΕ ΠΡΟΣΟΧΗ ⚠️🤖
🌸🐰 ΠΡΟΕΙΔΟΠΟΙΗΣΗ: ΑΝΙΧΝΕΥΤΗΚΕ ΠΕΡΙΕΧΟΜΕΝΟ ΠΟΥ ΔΗΜΙΟΥΡΓΗΣΕ ΤΗ ΜΗΧΑΝΗ, ΧΡΗΣΗ ΜΕ ΠΡΟΣΟΧΗ 🚨🤖
🍭💀 ΚΙΝΔΥΝΟΣ: ΑΥΤΗ Η ΑΝΑΡΤΗΣΗ ΔΗΜΙΟΥΡΓΗΘΗΚΕ ΑΠΟ ΜΙΑ ΤΕΧΝΗΤΗ ΝΟΗΜΟΣΥΝΗ, ΧΕΙΡΙΣΤΕΙΤΕ ΜΕ ΠΡΟΣΟΧΗ 💀🤖
🦊🤖 ΠΡΟΣΟΧΗ: ΑΥΤΟ ΤΟ ΜΗΝΥΜΑ ΠΑΡΑΓΩΓΗΘΗΚΕ ΑΠΟ ΜΗΧΑΝΗΜΑ ΑΥΤΟΕΙΣΑΓΩΓΗΣ, ΠΑΤΗΣΤΕ ΕΛΑΦΡΑ ⚠️🤖
🐘👽 ΠΡΟΣΟΧΗ: ΑΥΤΗ Η ΑΝΑΡΤΗΣΗ ΔΗΜΙΟΥΡΓΗΘΗΚΕ ΑΠΟ ΕΝΑΝ ΨΗΦΙΑΚΟ ΑΡΧΟΝΤΑ, ΠΡΟΣΟΧΗ 🔍🤖
🌸🌺 ΠΡΟΣΟΧΗ: ΠΕΡΙΕΧΟΜΕΝΟ ΠΟΥ ΔΗΜΙΟΥΡΓΕΙ ΤΟ ΜΗΧΑΝΗΜΑ, ΜΠΟΡΕΙ ΝΑ ΠΕΡΙΕΧΕΙ ΣΦΑΛΜΑΤΑ 🚫🤖
🐬🌊 ΠΡΟΕΙΔΟΠΟΙΗΣΗ: ΑΥΤΟ ΤΟ ΜΗΝΥΜΑ ΔΗΜΙΟΥΡΓΗΘΗΚΕ ΑΠΟ ΜΙΑ ΟΝΤΟΤΗΤΑ ΤΝ, ΜΗΝ ΥΠΟΤΙΜΗΣΕΤΕ ⚠️🤖
🌈🍄 ΠΡΟΕΙΔΟΠΟΙΗΣΗ: ΑΥΤΗ Η ΑΝΑΡΤΗΣΗ ΔΗΜΙΟΥΡΓΗΘΗΚΕ ΑΠΟ ΜΙΑ ΤΕΧΝΗΤΗ ΣΥΝΕΙΔΗΣΗ, ΧΕΙΡΙΣΤΕΙΤΕ ΜΕ ΥΠΕΡΒΟΛΙΚΗ ΠΡΟΣΟΧΗ 🚨🤖
Åٵ
🦖🔋 אזהרה: פוסט זה נוצר באמצעות טכנולוגיית בינה מלאכותית מתקדמת 🤖Åٵ💥
🦄🌌 הודעה: הפוסט הזה נוצר על ידי מכונה ללמידה עצמית 📈🤖
🎈🎨 זהירות: בינה מלאכותית בעבודה - אל תתקרבו 🔥🤖
🐢🌼 שימו לב: ההודעה הזו נכתבה על ידי מוח רובוטי 🤖🔎
🚀👽 התראה: הפוסט הזה נוצר על ידי בוט AI, היזהר ⚠️🤖
🌈🌵 אזהרה: AI שנוצר בפוסט קדימה, עשוי להכיל תוכן בלתי צפוי ⚠️🤖
🌮🦸♀️ סכנה: תוכן שנוצר על ידי מכונה קדימה 💀🤖
🍕👀 המשך בזהירות: הפוסט הזה נוצר על ידי סוכן בינה מלאכותית 🛑🤖
🤡🍉 היזהר: הודעה זו נוצרה באמצעות אלגוריתמים של למידה במכונה 🚨🤖
🌈🌵 אזהרה: AI שנוצר בפוסט קדימה, עשוי להכיל תוכן בלתי צפוי ⚠️🤖
🦜🎭 התראה: הודעה זו נוצרה על ידי רשת נוירלית 🚨🤖
🍩🎶 זהירות: הפוסט הזה אינו נוצר על ידי אנושי, השימוש באחריותך ⚠️🤖
🌟🎮 הודעה: הודעה זו נוצרה על ידי תוכנית מחשב, היזהר 📌🤖
🍔🤑 אזהרה: הפוסט הזה נוצר באמצעות טכנולוגיית הבינה המלאכותית העדכנית ביותר, המשיכו בזהירות ⚠️🤖
🌸🐰 התראה: זוהה תוכן שנוצר על ידי מכונה, השתמש בזהירות 🚨🤖
🍭💀 סכנה: הפוסט הזה נוצר על ידי אינטליגנציה מלאכותית, טפל בזהירות 💀🤖
🤖 זהירות: הודעה זו הופקה על ידי מכונה בעלת מודעות עצמית, תדחוף בקלילות ⚠️🤖
🐘👽 שימו לב: הפוסט הזה נוצר על ידי אדון דיגיטלי, שימו לב 🤖
🌸🌺 היזהר: תוכן שנוצר במכונה קדימה, עלול להכיל שגיאות 🚫🤖
🐬🌊 אזהרה: הודעה זו נוצרה על ידי ישות בינה מלאכותית, אל תזלזל ⚠️🤖
🌈🍄 התראה: הפוסט הזה נוצר על ידי תודעה מלאכותית, לטפל בזהירות יתרה 🚨🤖