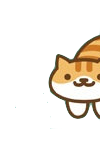

User Controls
I have single-handedly condensed all of language into consisting of 4 subsets.
-
2017-09-24 at 10:46 PM UTC
Originally posted by matrix i dunno but i'll invent one right now
Ga'cebp - tree
Ufifu - hand
Gofifed - sock
this language is based on colors and qualities of objects to make the syllables of the word to create an integrated framework of seeing and speaking
Ga'cebp
Ga- green color, there are a few colors that have a name that starts with G, so green is abbreviated and assigned the first vowel a with this mark '
E- connector vowel, used to construct the words out of qualities, this vowel is exempt from being assigned to abbreviations
C- cloud, Ga-C-Bp, so green color, cloud (shape property)
Bp- brown pole
so color + shape makes one syllable that describes an object, then the vowel e is used to connect these properties
(color + shape) green + cloud, brown + pole
(green + cloud) + (brown + pole)
(green + cloud) E (brown + pole)
ga'c + e + bp = ga'cebp
i can keep going with all of the examples if you want
2 days later, this thread sophie tries to pass off as his own
i am apex genius -
2017-09-24 at 10:48 PM UTC
Originally posted by Lanny It's an interesting question, like could we use ML approaches to generate a formal grammar? I don't know, in some sense techniques like Markov models in language tasks do produce a sort of grammar, they work for recognition and then you can turn them around and use them for generative tasks. So it is a kind of grammar, just not a great one in terms of mirroring natural languages (but to be fair the state of the art uses more sophisticated models).
But I think it turns into a kind of curve fitting issue, many grammars can produce a training set, how do we know which one was used? Like let's say I invent a language that is defined by its first order markov property: sentences are constructed by taking an existing sentence in the language and adding one more word depending only on the last word. And then I train a markov chain by feeding it a large number of sentences from this language. The "grammar" represented the trained model has might be able to produce every sentence in the language but it's not necessarily the same grammar as was used to train it. And we can think of all kinds of way to make the source grammar trick the model, maybe the grammar doesn't allow for sentences longer than N words but the model will never know that and produce illegally long sentences. That's an example specific to the markov model but I can't think of one that doesn't have the same issue. There's also Gold's theorem which formally rules out the possibility of language learning without negative input (someone saying "this isn't a valid sentence").
The philosophical response standard among the orthodoxy is that if you make a computer that can produce well formed sentences but it's not using the same grammar as humans, or which fails in edge cases, then it doesn't really matter. Humans produce ungrammatical sentences, and it's at least asking if all the speakers of the same language really do have the same mental grammar or if everyone's model of language be internally different but externally similar. And that's fair, if you take the goal of AI research to be the production of systems like chatbots and spell checkers and such then it really is a non-issue. But in my opinion the really important output of the AI project is not these systems but rather a greater understanding of human cognition. That's not a super popular opinion (although it is represented as a minority in the literature) but we kind of have a lot of human intelligence, 8 billion people, society isn't going to collapse if we have to employ some of them in language tasks, language skills are abundant and cheap. But insight into the nature of language itself, something this is a substrate of thought, that we all use easily every day but can't explain (we can identify qualities like "wordiness" or "bold writing" or "awkward wording" but can't really explain them intellectually), is fascinating.
try harder lol -
2017-09-24 at 10:52 PM UTCThe following users say it would be alright if the author of this post didn't die in a fire!
-
2017-09-24 at 10:55 PM UTC
Originally posted by Lanny It's an interesting question, like could we use ML approaches to generate a formal grammar? I don't know, in some sense techniques like Markov models in language tasks do produce a sort of grammar, they work for recognition and then you can turn them around and use them for generative tasks. So it is a kind of grammar, just not a great one in terms of mirroring natural languages (but to be fair the state of the art uses more sophisticated models).
But I think it turns into a kind of curve fitting issue, many grammars can produce a training set, how do we know which one was used? Like let's say I invent a language that is defined by its first order markov property: sentences are constructed by taking an existing sentence in the language and adding one more word depending only on the last word. And then I train a markov chain by feeding it a large number of sentences from this language. The "grammar" represented the trained model has might be able to produce every sentence in the language but it's not necessarily the same grammar as was used to train it. And we can think of all kinds of way to make the source grammar trick the model, maybe the grammar doesn't allow for sentences longer than N words but the model will never know that and produce illegally long sentences. That's an example specific to the markov model but I can't think of one that doesn't have the same issue. There's also Gold's theorem which formally rules out the possibility of language learning without negative input (someone saying "this isn't a valid sentence").
The philosophical response standard among the orthodoxy is that if you make a computer that can produce well formed sentences but it's not using the same grammar as humans, or which fails in edge cases, then it doesn't really matter. Humans produce ungrammatical sentences, and it's at least asking if all the speakers of the same language really do have the same mental grammar or if everyone's model of language be internally different but externally similar. And that's fair, if you take the goal of AI research to be the production of systems like chatbots and spell checkers and such then it really is a non-issue. But in my opinion the really important output of the AI project is not these systems but rather a greater understanding of human cognition. That's not a super popular opinion (although it is represented as a minority in the literature) but we kind of have a lot of human intelligence, 8 billion people, society isn't going to collapse if we have to employ some of them in language tasks, language skills are abundant and cheap. But insight into the nature of language itself, something this is a substrate of thought, that we all use easily every day but can't explain (we can identify qualities like "wordiness" or "bold writing" or "awkward wording" but can't really explain them intellectually), is fascinating.
Very interesting. Again, thanks for the words. -
2017-09-24 at 11:01 PM UTC
-
2017-09-24 at 11:03 PM UTCdegredation
-
2017-09-25 at 3:10 AM UTCIf you ever use the phrase "single handedly" without my permission ever again I will start making threats
-
2017-09-25 at 3:11 AM UTCtl dr looolool
-
2017-09-25 at 3:21 AM UTC
-
2017-09-25 at 3:25 AM UTC
-
2017-09-25 at 3:31 AM UTC
-
2017-09-25 at 3:31 AM UTCAutistic retarded faggot
-
2017-09-25 at 3:31 AM UTCSploo is an autistic retarded FAGG 00OT
-
2017-09-25 at 3:50 AM UTC
-
2017-09-25 at 3:53 AM UTCBy your own description , state and concepts are mutually the same?
-
2017-09-25 at 7:45 AM UTCThe following users say it would be alright if the author of this post didn't die in a fire!
-
2017-09-25 at 1:37 PM UTCOkay, but what are "concepts, locations, states, and times" in terms of concept/location/state/time? Are they all concepts? Then don't we just communicate through concepts?
-
2017-09-25 at 6:31 PM UTC
-
2017-09-25 at 8:24 PM UTC
-
2017-09-27 at 2:19 AM UTCbali chadhogay